Will AI Agents Replace Architects and Better Empower Enterprise Changemakers?
The fifth and final part of our blog series, “Generative AI and Enterprise Architecture,” by Ed Granger and Ardoq’s Chief Enterprise Architect Jason Baragry, touches on how AI can empower changemakers, potentially providing personalized and contextualized insights on demand.
This is the final part of this blog series on AI and EA, which provides an in-depth perspective on how generative AI will reshape EA as we know it. To catch up on the earlier parts of the series:
- Generative AI and Enterprise Architecture: Impact on Enterprise Complexity
- Generative AI and Enterprise Architecture: Modeling the Enterprise
- Generative AI and Enterprise Architecture: Roadmapping the Future
- Generative AI and Enterprise Architecture: Driving Business Outcomes
Axiom Five: EA Must Be Close to the Changemakers
For a few years now, technology analysts Gartner have been promoting their concept of ‘EA as an internal management consultancy’ — repositioning the role of the architect as a trusted advisor to enterprise changemakers.
This shift recognizes a core truth: As technology platforms have become increasingly commoditized, there are diminishing returns in EA’s original mission of technology standardization.
However, there’s great potential in leveraging EA’s holistic mindset — a byproduct of that same engineering background — to inform strategic business planning.
Now, it’s questionable whether, in practice, EA teams have succeeded in gaining a voice at the top table. But in many cases, they have shifted — either up or out into the technology change frontline, becoming embedded into product and project teams.
In either case, the principle holds: To do the most good, enterprise architecture must be close to where change happens.
So this is Axiom Number Five.
Impact is Proportional to Mass
It’s sad to say, but in many cases, enterprise architecture teams’ actual impact on organizational transformation has fallen short of their ambitions.
Of course, there are many success stories like those recognized annually in Forrester’s Enterprise Architecture Awards. They demonstrate how architecture teams can have an outsized impact on transformation relative to their size.
But the keyword here is “ambitions.” We architects should hold ourselves to very high standards because (at the risk of sounding grandiose) Enterprise Architecture’s ambition is to design the enterprise.
Okay — maybe not all of it, and certainly not all at once. But the ultimate point of a holistic organizational change practice must be to effect holistic organizational change. This is our hope as we compile our capability models and reference architectures, build and analyze application or process portfolios, and roadmap towards a better future.
There are several reasons why we may fall short, some of which we discussed in earlier parts of this blog series, like an overly academic focus or an inability to articulate business value.
However, a critical one we haven’t yet addressed is that a prime reason why many EA teams haven’t had a bigger impact is they don’t have the numbers.
EA teams can vary wildly in size, from as small as three to as big as a hundred. But in nearly every organization, they’re vastly outnumbered by the mass ranks of product managers, designers, developers, DevOps engineers, and the whole machinery of technology change. It’s this basic disparity in bandwidth that ultimately makes Enterprise Architecture’s job so challenging.
Architecture amplifiers include reference models, governance forums, architecture-as-code, and the good old (and too easily ignored) enterprise architecture portal.
But while defining and publishing those processes and artifacts is one thing, actually getting them embedded into frontline change has often proven a lot harder. EA team outputs require interpretation and contextualization to be useful in individual change initiatives. Unfortunately, that’s often a manual process that relies on available architecture bandwidth to do the interpreting and contextualizing.
Leveraging Generative AI to Shift from Mass Artifacts to Personalized Experiences
Generative AI promises a UX revolution for EA, transforming how EA engages with its audience by making architecture analysis and guidance both personal and contextual.
If that sounds like a stretch, it’s probably more of an imaginative leap than a technical one.
Experienced architects, more used to thinking about mass-consumer artifacts like standards or patterns or high-level analysis and recommendations to executives, may struggle to imagine how architecture can be used to build personalized user experiences for boots-on-the-ground changemakers — changemakers who are typically unaware of or ambivalent about Enterprise Architecture’s role.
However, Enterprise Architecture actually has a lot to offer them.
All change happens in the context of a complex enterprise ecosystem, one that most people struggle to navigate. Enterprise Architects are experienced in using models to understand the context of change to support a whole range of missions. For example:
- Understanding process impacts
- Flagging potential compliance issues
- Highlighting parallel projects as the basis for collaboration
- Recommending collaborators and communities of interest
- Recommending technology stacks and patterns to accelerate implementation
- Building in security by design
How might this work technically? Well, in broad terms, we need three categories of information, all of which could form part of a RAG (Retrieval Augmented Generation) pipeline into an LLM.
- The individual’s relationship with the current business/technology/information landscape (for example, the process they own or the application interface they’re modifying).
- Architecture models to inform the context they’re working within (e.g. related processes or applications) for impact analysis.
- Architecture guidance relevant to the scope of their changes (e.g. data residency requirements or messaging encryption standards).
As we saw in Part 3 of this series on roadmapping, LLMs are well able to associate relevant information based on their training, even in the absence of a formal reasoning capability. This is enough to start automating the process of interpretation and contextualization.
So imagine if technology changemakers each had a personalized architect — a ‘good angel’ looking over their shoulder — highlighting and guiding them through the labyrinth of enterprise change by using the models and guidance EA produces to inform its interactions.
What impact might that have on the EA team’s ability to affect the course of transformation? It could be profound.
The Rise of the Machines?
If that all sounds just a little utopian and too good to be true, maybe it is. Because there’s potentially a big fly in our ointment.
We’re not actually sure who those changemakers will be.
We’re not talking about the C-suite here. It’s a safe bet that, long after the enterprise has been reduced to rows upon rows of blinking servers, there will still be executives of flesh and blood occupying the top floor.
Even if its immediate effects have almost certainly been overstated, generative AI has the potential to unleash major demographic shifts in the workforce. For architects, this means that in the longer term, who they collaborate with and what they collaborate on could change dramatically.
Each wave of enterprise technology, from the mainframe, the LAN, and the internet to SaaS and cloud, has driven a steady expansion in the number of technology roles. Job titles like ‘UX Writer’ or ‘DevOps Engineer’ just didn’t exist ten years ago. Now, they’re commonplace.
We might call them machine-whisperers — people who know either how to speak the language of the machines or to translate the machines’ language into our own.
Over the past four decades, more and more people have taught themselves how to ‘speak machine’ to meet an ever-expanding demand for digital products and services.
But what now that the machines speak our language?
Will We Need Humans At All?
When a one-line prompt can generate lines of code or pages of content, something fundamental has changed about the economics of building and running information systems.
Over the past 12 months, there have been some pretty stark assessments of AI's impact on jobs, not only in the tech sector but across all kinds of knowledge work.
Goldman Sachs estimated that generative AI could destroy north of 300 million jobs globally in the coming years, while the UK Government’s own analysis identifies workforce disruption as one of the top five societal risks from AI.
Once picked up and amplified by news algorithms, these predictions can trigger a sense of impending doom in the white-collar workforce. But there’s also a lot of vested interest powering that hype, so we need to take a more objective, evidence-led view. So far, the signals are decidedly mixed.
On the one hand, there are early indicators that the impacts are already being felt in some sectors, particularly those involved in content creation; equally, there are early signs of new generative AI-specialized roles like Prompt Engineer or GenAI Technologist trickling into the jobs market.
But that trickle isn’t yet a flood. And there are also many voices sceptical of wholesale shifts in the jobs market.
Again, we need some kind of lodestone to ground our reasoning. A good one is to follow the money and look where the investment is going.
One indicator is the increasing adoption of ‘Copilot’ patterns of generative AI integration in existing enterprise software, exemplified by Microsoft 365’s or GitHub’s respective Copilots. Here, the clear pattern is resource augmentation, not replacement.
With the existing global enterprise SaaS market worth an estimated $197B in 2023 and the long-term model for the monetization of generative AI still emerging, it’s not hard to see why Big Tech is not in a rush to take the humans out of the loop.
Likewise, if we dig a little deeper into those Goldman numbers, we can see an estimated 29% of technology tasks could be performed by generative AI. That’s a lot — but it still leaves a substantial margin that depends primarily on human resources.
So, in the near term, at least, the enterprise software market is betting on personalized agents as productivity enhancers for existing changemakers.
There’ll probably be humans on the shop floor for a little while yet.
Autonomous AI Agents of Change
There are also fundamental questions that need to be answered before we can talk about any kind of fully AI-driven organization.
Integration, Compliance, and Risk
How does generative AI integrate with highly regulated financial control systems? How does it integrate into a supply chain? What are the liability risks? In fact, how do LLMs integrate with the whole transactional machinery of the enterprise? There’s also the matter of generative AI’s 90% accuracy rate to contend with.
Amara’s Law states that we tend to overestimate the effect of new technology in the short run and underestimate it in the long run.
In the short term, we need to remember we’ve spent 40 years investing in transaction processes, code bases, databases, logistical, audit and control systems that, however well or badly designed, nonetheless are the operating enterprise.
Replacing your marketing press releases with AI-generated content is one thing; unpicking your sales ledger from live operational processes is quite another.
Will the Architect Be Redundant in the Future Enterprise?
However, in the long term, we can already see glimpses of what the next generation of enterprise could look like.
Autonomous AI agents are already here. These solutions use LLMs as a foundation from which to build goal-seeking artificial actors that can autonomously break down and execute tasks, either individually or in collaboration with other AI agents, with limited human intervention or supervision.
Take Microsoft’s AutoGen, where teams of agents interact to execute a process. Here, the orchestration of the traditional layers of architecture — application, data, and processes —is subsumed into an opaque generative AI workflow.
For these scenarios, drawing a Visio map to show how processes span architecture layers seems pretty pointless. It neither specifies nor accurately represents the process execution.
So, is the architect’s role redundant in the autonomous enterprise?
We don’t think so. Even in this strange world of teams of artificial agents, the enterprise architect still has a role to play because one of the most compelling questions for any notional agent-driven enterprise is how to align intent.
As we’ve already seen, intent is not AI’s strong point. But if the enterprise is to cede control of its day-to-day operations to these autonomous agents, then it’s essential their outcomes are consistent with those of the organization.
The Challenge of Coherency and Control
How to match autonomy with accountability in AI agents is an emerging area of both research and governance. In its broadest sweep, it includes ethical and legal considerations, with both governments and Big Tech themselves starting to focus now on control mechanisms for agentic AI systems.
These top-down controls represent a generic baseline set of constraints. They’re broadly equivalent to HR policies that ensure that employees don’t break the law, adhere to acceptable codes of conduct, respect organizational confidentiality, etc.
In Enterprise Architecture’s case, we’re talking about something more specialized: How do we ensure that autonomous agents actually behave like a coherent organization and not as a series of disconnected units? How do we align and orchestrate them to deliver the enterprise’s shared outcomes, whether financial, societal, or environmental?
One approach might be to simply outsource this job to another AI agent operating at a different level. ‘It’s turtles all the way down!’
Still, strategy and policy — the ultimate source of organizational coherence and conscience — will probably be the last things to be automated. If nothing else, accountability to investors and customers probably demands it. So, the governance patterns we’re seeing emerge clearly stress the importance of human-in-the-loop safeguards.
These need to be architected. While it's early days yet (a recurring theme in this series), we’re starting to see candidate reference architectures and frameworks for aligning the behavior of autonomous agents at different levels of grain: Task, process, organizational, and societal outcomes.
What’s striking about some of these models is their similarity to conventional Enterprise Architecture frameworks in the way that they map detailed operational activity up to high-level strategic intent. For example, the principles of abstracting processes and processing resources up to business capabilities and aligning them with organizational strategy models. There are clear echoes of this in these emerging autonomous agent architectures and governance frameworks.
If that is the case, then it suggests that Enterprise Architecture addresses fundamental problems of system complexity and coherence — not just in human systems, but in any system.
So, as long as that remains true, enterprises will still need architects.
Putting it All Together: Building for the Future
When ChatGPT, DALL-E, Midjourney, et al. broke out over a year ago, it triggered a collective state of future shock. The aftershocks rumble on to this day, triggered by each new generative AI product announcement (at the time of writing, OpenAI’s Sora text-to-video model has just debuted to widespread astonishment).
For architects, that future shock can be magnified twofold. It’s not only the impressive, almost alien capabilities of this new technology that challenge us as technology consumers. As technology builders, it also upends many conventional assumptions around information processing — assumptions that Enterprise Architecture is built on.
As the shock subsides and we begin to integrate AI into our work and lives, our questions become more specific and more targeted: Not so much ‘how does it even work?’ as ‘how does it work here?’
Hopefully, this series of articles on generative AI and EA has gone some of the way towards answering that question — or, at the very least, shaping that conversation. While futurology is notoriously unreliable, it’s never more so than when it takes only a passive role in shaping that future.
On a personal note as Enterprise Architects, now that we’re deeper into it and learning daily, we’re grateful for this future shock. It represents a gift we’re not often given: A chance to step back and reimagine.
So we should ask ourselves, what kind of Enterprise Architecture will we pass on to the next generation of EAs? These are people who are just starting the journey of looking beyond the borders of their screens and wondering how the whole organization hangs together, people who grew up with smartphones and social media, and for whom vector databases and LLMs were the first technologies they got under the hood of, not the latest.
Because if we truly are architects and strategists, then that's the future we need to be building for.
This was the last of our five-part blog series, deep-diving into generative AI's promise and potential problems in Enterprise Architecture. All previous parts are linked below.
If you're hungry for more content on AI and EA, watch our webinar on-demand: Enhancing Enterprise Architecture with AI: An Ardoq Perspective
This blog series has been co-authored with Ardoq’s Chief Enterprise Architect, Jason Baragry.
- Generative AI and EA Series Generative AI and EA: Impact on Enterprise Complexity Generative AI and EA: Modeling the Enterprise Generative AI and EA: Roadmapping the Future Generative AI and EA: Driving Business Outcomes
- Blog Posts Leveraging AI for Strategic Enterprise Planning The Advantages of AI for Digital Transformation
- Webinars Enhancing Enterprise Architecture With AI | 29 February 2024 | Recording The Future Is Now: How AI Will Power EA | 6 June 2023
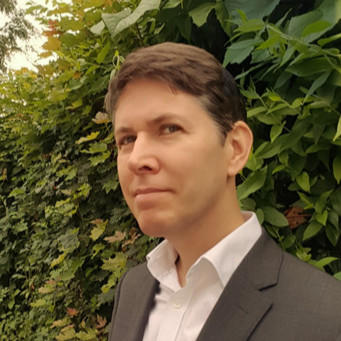